Bridge - High-Resolution Digital Photography
Target of Investigation
High-resolution digital photography can be used as a vision-based nondestructive evaluation technique for bridge inspections, providing image data that support inspection reports, develops three-dimensional (3D) digital model reconstruction for bridge information modeling and documents, and detects and maps surface defects (e.g., cracks). Figure 1 shows an example of conducting vision-based bridge inspection for the Ticonic Bridge in Maine. The high-resolution digital photography can also be used for other engineering applications, including land surveying and emergency management of flooding.(1) This web page primarily focuses on using high‑resolution digital photography for surface crack detection.
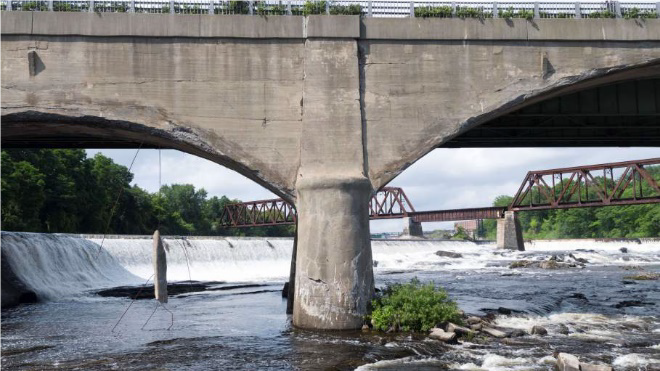
© 2020 VHB.®
Description
High-resolution digital photography refers to the capture of digital images with a large number of pixels, resulting in a high level of visual details. This capture is typically achieved by using high‑definition (HD) digital cameras with advanced image sensors and lenses technology. Now high-resolution digital photography for vision-based bridge inspections mainly uses unmanned aerial systems (UAS) equipped with HD digital cameras. Because UAS has the capability of collecting HD image data in challenging environments with limited traffic control, HD imaging through UAS can improve efficiency, enhance safety, and lower the economic costs of bridge inspections compared to manual visual inspections by inspectors. The collected HD image data can support inspection reports, develop 3D-digital model reconstruction, and diagnose surface defects (e.g., cracks).
The creation of a 3D-digital model provides bridge owners with many advantages, including creating a structural visualization in the absence of original drawings, supplementing essential information for numerical modeling, which can be used alone or in combination with other inspection data, and providing a data archive for postinspection review. Surface defect mapping provides useful information about the location and severity of surface defects across structural surfaces. The mapping results can be used as evidence for executing additional indepth analysis or inspection. For more information about the use of UAS to support bridge inspections, refer to a Federal Highway Administration (FHWA) report and TechBriefs. (See references 2–5.)
Physical Principle
Digital cameras capture images through an optical system that uses a lens with a variable diagram to focus light onto an electronic image-pickup device. Because surface defects (e.g., cracks) present different visual appearances than surrounding areas, surface defects are likely identifiable through visual examination or image analysis.
Data Acquisition
Data acquisition involves collecting image data from digital cameras. Various features of the camera and its operations may influence the quality of the images. Based on laboratory results from an FHWA-sponsored research project, the following sensor specification and operational practices for using UAS digital cameras are recommended:(4)
- Sensor resolution should be at least 12 megapixels.
- Automatic sensor setting is sufficient.
- Maintain a minimum standoff distance of 5 ft.
- Optical zoom capability is a plus but not necessary.
- Augmented lighting can improve image quality in low-light conditions.
Data Processing
Crack detection can be manually implemented through visual examination, which is labor intensive and inefficient. Automatic crack detection is achievable through image processing techniques. Sobel and Canny edge detectors are examples of two edge detection algorithms available.(5,6) With recent advancements in machine learning and the availability of large-scale training image databases, using deep-learning algorithms for crack detection is more efficient and accurate, and thus, deep learning has become popular and dominant in recent years. The convolutional neural network is the primary deep-learning model that yields high accuracy for crack detection.(7) For more information about using deep learning for crack detection, see an early work by a group of researchers from the Massachusetts Institute of Technology and Canada, and a recent report from an FHWA-sponsored research project.(8)
Data Interpretation
Crack detection results are usually visually intuitive. All visible cracks should be properly noted in bridge inspections.(9,10)
Advantages
- Identify surface defects through visual examination.
- Achieve limited traffic control with UAS.
- Hold potential for automatic crack detection with advanced image processing algorithms.
- Enable data storage for further use (e.g., 3D-digital model reconstruction and postinspection review).
Limitations
- Manual visual examination can be subject to performance fatigue of inspectors.
- Special specifications are required for the camera and its operation.
- Moderate to significant expertise and training are required for developing automatic crack detection.
References
- FHWA. n.d. “Unmanned Ariel Systems” (web page). https://www.fhwa.dot.gov/uas/library.cfm, last accessed February 22, 2024.
- Neubauer, K., E. Bullard, and R. Blunt. 2021. Collection of Data with Unmanned Aerial Systems (UAS) for Bridge Inspection and Construction Inspection. Report No. FHWA‑HRT-21-086. Washington, DC: Federal Highway Administration. http://www.fhwa.dot.gov/publications/research/infrastructure/structures/bridge/21086/21086.pdf, last accessed February 22, 2024.
- Cheyne, D., B. Merrill, and J. Boggs. 2019. Use of Small Unmanned Aerial Systems for Bridge Inspection. Publication No. FHWA-HIF-19-056. Washington, DC: Federal Highway Administration. https://www.fhwa.dot.gov/uas/resources/hif19056.pdf, last accessed February 22, 2024.
- Azari, H. 2021. Controlled-Environment Testing of UAS Digital Camera Sensor Specifications and Operational Parameters for Bridge Safety Inspections. Publication No. FHWA-HRT-21-060. Washington, DC: Federal Highway Administration. https://highways.dot.gov/media/1891, last accessed February 22, 2024.
- Abdel-Qader, I., O. Abudayyeh, and M. E. Kelly. 2003 “Analysis of Edge-Detection Techniques for Crack Identification in Bridges.” Journal of Computing in Civil Engineering 17, no. 4: 255–263.
- Canny, J. F. 1986. “A Computational Approach to Edge Detection.” IEEE Transactions on Pattern Analysis and Machine Intelligence 8, no 6: 679–698.
- Azari, H. 2021. Effective Practices for Routine Bridge Inspections Using Unmanned Aerial Systems. Publication No. FHWA-HRT-21-083. Washington, DC: Federal Highway Administration. www.fhwa.dot.gov/publications/research/infrastructure/structures/bridge/21083/21083.pdf, last accessed February 22, 2024.
- Cha, Y.-J., W. Choi, and O. Buyukozturk. 2017. “Deep Learning-Based Crack Damage Detection Using Convolutional Neural Networks.” Computer-Aided Civil and Infrastructure Engineering 32, no. 5: 361–378.
- Alavi, A. H., Q. Zhang, S. Babanajad, F. Moon, J. Braley, and N. Gucunski. 2021. Improving Bridge Assessment Through the Integration of Conventional Visual Inspection, Non-Destructive Evaluation, and Structural Health Monitoring Data. Report No. FHWA-PA-2021-012-IRISE WO 01. Pittsburgh, PA: University of Pittsburgh. https://issuu.com/pittswanson/docs/bridge_assessment_final_report_irise, last accessed February 23, 2024.
- Ryan, T. W., J. E. Mann, Z. M. Chill, and B. T. Ott. 2002. Bridge Inspector’s Reference Manual. Report No. FHWA NHI 12-050. Washington, DC: Federal Highway Administration. https://www.dot.state.mn.us/bridge/pdf/insp/birm/birmchapt0-cover.pdf, last accessed February 23, 2024.